The Role of Large Language Models (LLMs) in Developer Tools
Code editors are no longer just tools for writing and formatting text, they’re becoming interactive environments, capable of understanding intent, suggesting solutions, and even reasoning through complex tasks. In this blog, we’ll look at how LLMs are shaping the next generation of developer tools.
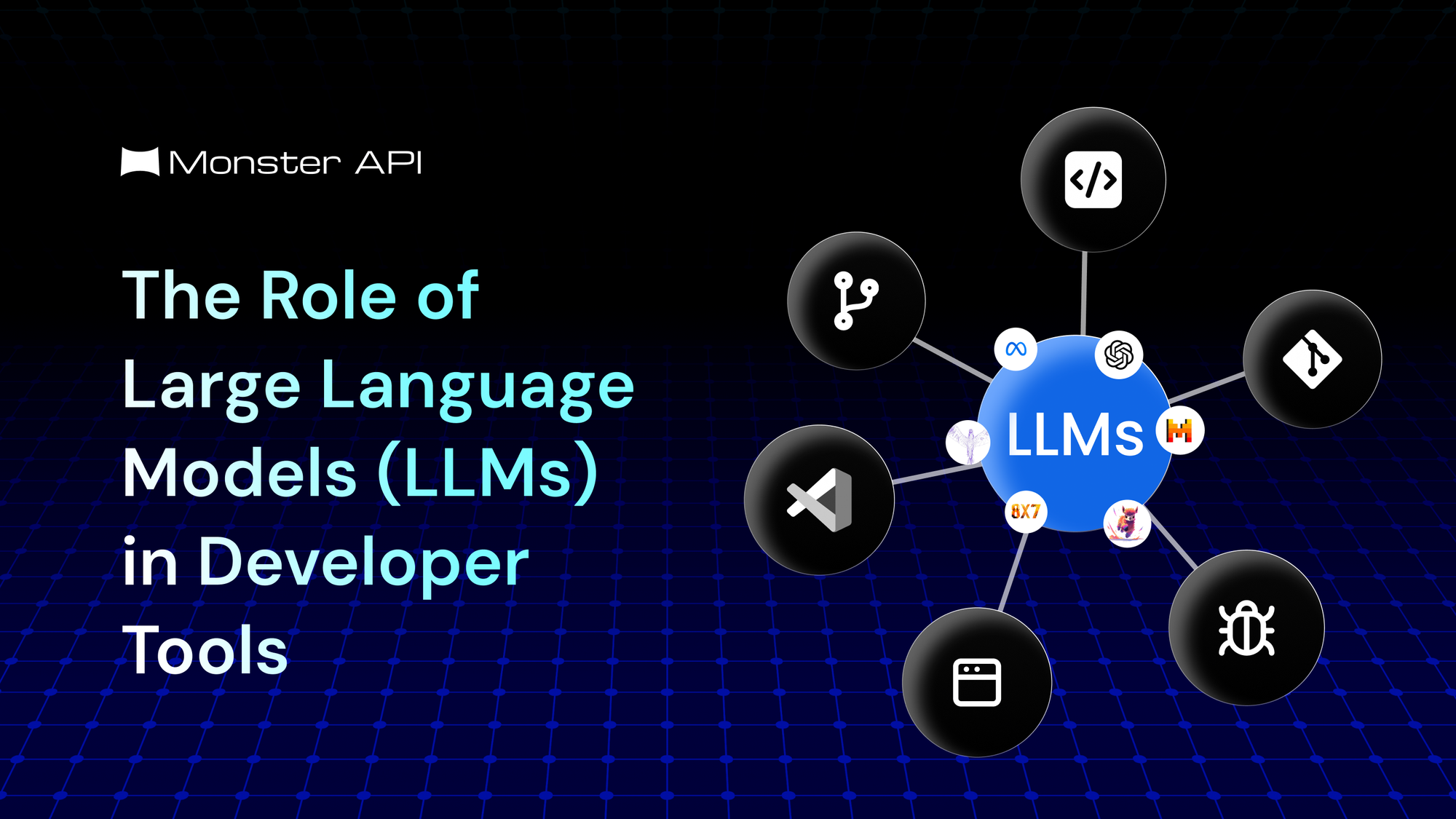
Introduction
Over the past few years, developer workflows have started to shift in a noticeable way. Code editors are no longer just tools for writing and formatting text — they’re becoming interactive environments, capable of understanding intent, suggesting solutions, and even reasoning through complex tasks.
This change is largely driven by the rise of Large Language Models (LLMs). Built on the same foundation that powers modern AI chatbots, these models are now being integrated into tools like Cursor, GitHub Copilot Workspace and Windsurf making them far more than just autocomplete engines. They're designed to assist with everything from writing code to reviewing it, generating tests, debugging, and even explaining unfamiliar codebases.
In this blog, we’ll look at how LLMs are shaping the next generation of developer tools. We'll break down what makes these tools different from traditional ones, how they actually work under the hood, the kinds of tasks they’re good at (and where they still fall short), and what this all means for developers moving forward.
What Are Developer Tools?
Developer tools, broadly speaking, are the systems and interfaces that assist programmers in writing, testing, analyzing, and maintaining code. These tools exist to simplify complex workflows, reduce human error, improve efficiency, and support collaboration across teams.
They range from the most basic utilities—like text editors and terminal emulators—to more specialized environments like Integrated Development Environments (IDEs), automated testing frameworks, and performance profilers.
The role of developer tools is not limited to writing code. They also support a wide range of tasks such as:
- Code organization and navigation
- Syntax highlighting and formatting
- Dependency and package management
- Version control integration
- Code linting and static analysis
- Debugging and runtime inspection
- Testing and test case management
- Documentation generation
- Continuous integration and deployment
As software systems have grown in complexity, the tooling around development has grown with them—moving from command-line utilities to highly customizable, full-stack environments that manage everything from code quality to deployment automation.
While these tools have become more powerful over time, their behavior has largely remained deterministic and rule-based—until recently.
Types of Traditional Developer Tools
Before Large Language Models entered the picture, developer workflows were already supported by a mature ecosystem of tools. These tools were designed to address specific stages of the software development lifecycle, from writing and testing code to building and shipping applications.
While implementations vary, most traditional developer tools fall into the following broad categories:
1. Code Editors and Integrated Development Environments (IDEs)
These are the core interfaces where most developers spend the bulk of their time. They offer features like:
- Syntax highlighting and code folding
- Project-wide search and navigation
- Basic autocomplete and refactoring tools
- Plugin ecosystems for extending language support
Examples: VS Code, IntelliJ IDEA, Eclipse, Sublime Text.
While some IDEs offer static code suggestions or pre-trained completion engines, these capabilities are typically rule-based or pattern-driven.
2. Linters and Static Analysis Tools
These tools inspect code without executing it, helping developers catch style violations, potential bugs, and anti-patterns early.
- Linting enforces formatting and coding conventions
- Static analyzers detect type mismatches, null references, unreachable code, etc.
Examples: ESLint, Pylint, SonarQube, Clang Static Analyzer.
Their logic is deterministic—driven by pre-defined rules or abstract syntax trees (ASTs), not adaptive models.
3. Compilers, Interpreters, and Build Tools
These tools handle the transformation of code into executable programs, managing dependencies, compiling assets, and coordinating build pipelines.
- Compilers translate high-level code into machine code
- Interpreters execute code directly
- Build systems coordinate compilation, linking, testing, and packaging
Examples: GCC, javac, Python interpreter, Webpack, Bazel, Gradle, Make.
While critical to dev workflows, these tools operate independently of the semantics or meaning behind the code being written.
4. Debugging and Profiling Tools
Debuggers help track the flow of program execution, inspect variables, and set breakpoints. Profilers help identify performance bottlenecks in running applications.
Examples: GDB, Chrome DevTools, PyCharm Debugger, Valgrind, perf, VisualVM.
These tools are tightly coupled to runtime behavior and require manual instrumentation or inspection by developers.
5. Testing Frameworks and CI/CD Pipelines
Testing frameworks support unit, integration, and end-to-end testing, while CI/CD systems automate test execution, linting, and deployment steps on every code push.
Examples: JUnit, pytest, Mocha, Selenium, Jenkins, GitHub Actions, CircleCI.
These tools focus on validating correctness and quality, often requiring developers to write specific tests or configuration files.
6. Documentation and API Tools
Documentation generators extract and format comments, docstrings, and API definitions into human-readable formats.
Examples: JSDoc, Doxygen, Sphinx, Swagger/OpenAPI.
They are useful for onboarding, maintaining standards, and making APIs easier to consume—but rely heavily on structured input.
These categories formed the backbone of developer workflows for decades. But despite their variety and power, most of them operate with no understanding of developer intent. They rely on predefined rules, static configurations, and hand-written logic. This is the gap that LLMs are now starting to fill.
The Emergence of LLM-Powered Developer Tools
The last few years have seen the introduction of a new generation of developer tools—ones that don’t just respond to keystrokes or execute predefined logic, but interpret intent, operate across entire codebases, and assist with tasks that traditionally required human judgment.
These tools are built around Large Language Models (LLMs), integrated not as side features but as core engines. Unlike traditional rule-based systems, they generate responses based on probabilistic reasoning, drawing from patterns learned across massive code and text corpora.
What sets these tools apart isn’t just their ability to autocomplete code. It’s their ability to:
- Understand natural language descriptions and translate them into working functions
- Analyze unfamiliar codebases and explain logic across multiple files
- Suggest refactors or improvements based on context, not just syntax
- Write tests, documentation, or summaries on demand
Rather than operating in isolated steps, these tools aim to support broader workflows: writing, reviewing, debugging, testing, and maintaining code—sometimes in a single interface, sometimes through conversational interactions, and often across multiple layers of abstraction.
They’re not merely smarter plugins. They represent a shift in how developers interact with code: moving from direct control to collaborative intent, where you describe what you want and the tool figures out how to get there.
Core Capabilities of LLM-Powered Developer Tools
What sets these tools apart isn’t just the presence of a language model—it’s how deeply the model is integrated into the developer workflow. Instead of acting as passive assistants, LLMs now operate as embedded systems capable of guiding entire development tasks from planning to execution.
In many of today’s LLM-powered environments, the coding experience starts with a prompt—not a file. You describe the problem or feature in plain language, and the system begins by outlining an approach, drafting function stubs, and in some cases, even creating file structures. This isn’t basic autocomplete—it’s collaborative authoring that extends across the entire codebase.
These tools offer several key capabilities that traditional environments simply couldn’t support:
1. Contextual Code Generation and Completion
Code suggestions no longer rely on just what’s visible in the current file. Instead, the LLM reads across multiple files, tracks dependencies, and adapts to the conventions and naming patterns of the project. This allows it to generate entire functions or modules that fit the existing structure naturally.
For example, when implementing a new feature, the system may suggest utility functions based on helper files elsewhere in the repo—or propose code that mirrors patterns it has identified across the entire codebase.
2. Natural Language to Code Workflows
One of the most visible shifts is the ability to describe what you want in plain English—and get working code in response. This could be as simple as writing, “Create a function to validate email addresses,” or as complex as defining a multi-step API integration.
Rather than relying on search or trial-and-error, the model interprets intent, plans the structure, and fills in the implementation—all within a conversational loop. You can accept, modify, or reject suggestions, and the system iterates accordingly.
3. Multi-File Reasoning
Modern LLM tools are capable of tracing logic across files, understanding how components interact, and even detecting bugs that span multiple modules. They treat the project as a unified system, not a collection of isolated files.
This allows developers to ask questions like “Where is this function called?” or “Why does this hook return null?” and receive answers that combine static analysis with learned reasoning patterns.
4. Automated Debugging and Fix Suggestions
When an error is encountered, the developer doesn’t need to jump into Stack Overflow or dig through logs. They can describe the issue, paste an error message, or highlight a failing test, and the model can propose likely causes and possible fixes—often in the correct syntax and project context.
The system doesn’t just explain what went wrong. It adapts the fix to the local code structure, following the idioms and libraries already in use.
5. Test Generation and Validation
Many tools now support automated test generation, either by analyzing existing functions or from written requirements. The LLM can propose test cases, edge conditions, and coverage improvements that might be overlooked in a manual review.
In some cases, it can also suggest which tests are likely to fail based on recent changes—helping developers catch regressions before they make it to CI.
6. Documentation and Code Explanation
From docstrings to full API documentation, these systems can summarize what a function does, what parameters it takes, and how it fits into the broader module. More importantly, they adapt their explanations based on the audience: technical summaries for developers, high-level overviews for non-technical stakeholders.
This is particularly helpful in onboarding contexts, where new team members can query the codebase conversationally and get contextual explanations in return.
7. Command and Tool Integration
Some systems go even further, allowing developers to interact with terminals, search files, run commands, and refactor code through natural language. The LLM acts not just as a code generator but as an orchestrator—bridging between the editor, CLI, search tools, and even external APIs.
In effect, these tools reshape the boundaries between writing, navigating, and executing code. They blur the line between human instruction and automated execution—creating workflows where entire tasks can be scoped, implemented, and validated without leaving the editor.
Real-World Examples
The integration of LLMs into developer tools has led to the emergence of advanced platforms that not only assist in code completion but also understand context, suggest improvements, and automate complex tasks. Let's explore three notable examples:
1. Cursor
Overview: Cursor is an AI-powered code editor developed by Anysphere Inc. It's a fork of Visual Studio Code (VS Code) augmented with advanced AI capabilities.
Key Technical Features:
- AI Autocompletion: Cursor utilizes LLMs to provide intelligent code autocompletion, predicting multi-line code snippets based on the current context.
- Smart Rewrites: The editor can suggest and apply multiple code edits simultaneously, facilitating efficient refactoring and error correction.
- Natural Language Editing: Developers can input instructions in plain English to modify code, allowing for intuitive code transformations.
- Codebase Understanding: Cursor indexes the entire codebase, enabling developers to query and navigate the code using natural language.
- Privacy and Security: Cursor offers a Privacy Mode where code is not stored remotely, ensuring data security. It is also SOC 2 certified.
2. GitHub Copilot Workspace
Overview: GitHub Copilot Workspace is an AI-native development environment designed to integrate AI assistance throughout the software development lifecycle. It allows developers to brainstorm, plan, build, test, and run code using natural language, streamlining the development process.
Key Technical Features
- Task-Centric Workflow: Development begins with a "task" — a natural language description of intent. Copilot Workspace supports tasks like solving issues, refining pull requests, and creating repositories from templates.
- Integrated Development Environment: The workspace includes a file explorer, integrated terminal, and supports building, running, and testing code directly within the environment.
- Version Control Integration: Copilot Workspace automatically versions the context and history of changes, allowing for easy creation of pull requests and collaboration.
- Multi-Model Support: Developers can choose between different AI models, including those from OpenAI, Anthropic, and Google, to best suit their tasks.
3. Windsurf
Overview: Windsurf, developed by Codeium, is an AI-native Integrated Development Environment (IDE) designed to keep developers in a "flow state" by integrating AI assistance throughout the development process.
Key Technical Features:
- Cascade: An agentic chatbot that collaborates with developers, understanding the entire codebase and assisting with complex tasks.
- AI Flows: Combines the capabilities of copilots and agents, allowing for seamless collaboration between the developer and AI.
- Supercomplete: Provides advanced code completion suggestions by tracking command history, clipboard, and previous actions.
- Integrated Terminal: An upgraded terminal experience that allows for executing commands and scripts directly within the IDE.
- Persistent Memory: Windsurf maintains context across sessions, remembering past interactions and decisions to provide relevant suggestions.
Each of these tools Cursor, GitHub Copilot Workspace, and Windsurf offers unique approaches to integrating AI into the development workflow.
How MonsterAPI Powers and Simplifies the Backend of Developer Tools
While tools like Cursor, GitHub Copilot Workspace, and Windsurf focus on developer experience and frontend orchestration, they all fundamentally rely on the ability to access, fine-tune, and scale LLMs behind the scenes. This is where platforms like MonsterAPI become critical.
MonsterAPI provides the infrastructure and tooling backend to power such LLM-enhanced environments—allowing teams to run, customize, and optimize models with speed and affordability.
Here’s a breakdown of how these developer tools (or teams building similar ones) can leverage MonsterAPI under the hood:
1. Low-Latency Model Inference for Interactive Code Sessions
Every code suggestion, documentation generation, or multi-file refactor in tools like Windsurf and Cursor is driven by real-time LLM inference. MonsterAPI supports high-speed, low-latency inference endpoints for popular open-source models like:
- Code-specialized LLMs: CodeLlama, DeepSeek Coder, Phi-2
- Instruction-tuned models: Mistral, Gemma
- Multimodal capabilities: For combining code+UI or docs+diagrams understanding
This allows teams to:
- Serve private or self-hosted LLMs via secured API endpoints
- Use multiport inference to support autocomplete, QA, summarization, and refactoring in parallel without collisions
- Integrate directly into VS Code extensions or web-based editors with a consistent API interface
Example: Cursor’s backend model endpoint could be routed through a MonsterAPI deployment of a fine-tuned CodeLlama 13B, with custom completion temperatures and token windows optimized for their editor UX.
2. One-Click Fine-Tuning for Custom Developer Experience
Tools like GitHub Copilot Workspace benefit immensely from domain-specific tuning—especially when assisting enterprise teams with private codebases, proprietary frameworks, or unique naming conventions.
MonsterAPI offers UI-based fine-tuning (no coding required), making it possible for product teams to:
- Upload internal datasets (code snippets, PR reviews, test cases, error logs)
- Generate synthetic data or use prompt-chaining for augmentation
- Fine-tune base LLMs on private corpora using LoRA or QLoRA techniques
- Deploy fine-tuned versions instantly to dedicated endpoints
Example: A company using Copilot-like tools internally can train a model on their own TypeScript + AWS Lambda patterns, then fine-tune it on MonsterAPI and connect it to their Windsurf environment. The result? Completions that mirror the company’s style guides and internal abstractions.
3. Scalable Deployment Infrastructure With Cost Efficiency
Behind every “Generate test,” “Fix this bug,” or “Explain this repo” action lies a series of LLM calls, each with its own token load and context requirements. Serving these models cost-effectively and reliably at scale is non-trivial.
MonsterAPI solves this by offering:
- On-demand GPU scaling: spin up high-memory GPUs only when needed
- Dedicated model hosting for enterprise SLAs
- Multi-model deployment under single interface (e.g., serve Phi-2 for inline edits, DeepSeekCoder for multi-file refactors)
- Prompt logging and monitoring dashboards to evaluate prompt performance over time
Example: Windsurf could route different agents (e.g., TestAgent, FixAgent, ExplainAgent) to different MonsterAPI models using multiport endpoints, optimizing for cost (small models for lightweight tasks, large ones for reasoning-heavy jobs).
4. Integration With Tooling Stacks (CLI, CI/CD, and Custom Agents)
Developer environments increasingly operate as full-stack agents, calling LLMs during CI pipelines, post-commit hooks, or CLI executions.
MonsterAPI supports integration with:
- Gradio-based tools for building debugging or review dashboards
- Webhooks and agent runners to trigger fine-tuned models from custom scripts
- CI pipelines (e.g., GitHub Actions) to test LLM predictions or auto-generate code comments
Example: A GitHub Copilot Workspace user clicks “Open a PR with explanation.” The action sends context to a MonsterAPI endpoint, where a custom model adds a rationale to the commit message and suggests reviewers based on code ownership history.
5. Model Experimentation Without Vendor Lock-In
Unlike closed tools that tie users to a single provider (e.g., OpenAI), MonsterAPI is model-agnostic. It supports both open-source and licensed models, enabling:
- Testing across different architectures (e.g., Gemma vs. Mistral vs. DeepSeek)
- Evaluating response quality with built-in A/B testing frameworks
- Rapid switching of backends without re-architecting the frontend
This gives teams the flexibility to optimize for accuracy, latency, or cost depending on task and user feedback.
Conclusion
Developer tools are evolving from rigid, rule-based systems to intelligent environments that understand code, context, and intent. With LLMs at their core, the new wave of tools doesn't just assist developers—they actively collaborate, adapting to workflows and scaling with complexity. But the effectiveness of these tools depends on more than their UI or features. Behind every intelligent suggestion or refactor is a model that needs to be fine-tuned, served, and scaled reliably. Platforms like MonsterAPI fill this critical gap—turning LLM-backed developer tools into practical, customizable, and production-ready systems. As this shift continues, the line between writing code and guiding intelligent systems will only get thinner.